DanceNet3D: Music Based Dance Generation with Parametric Motion Transformer
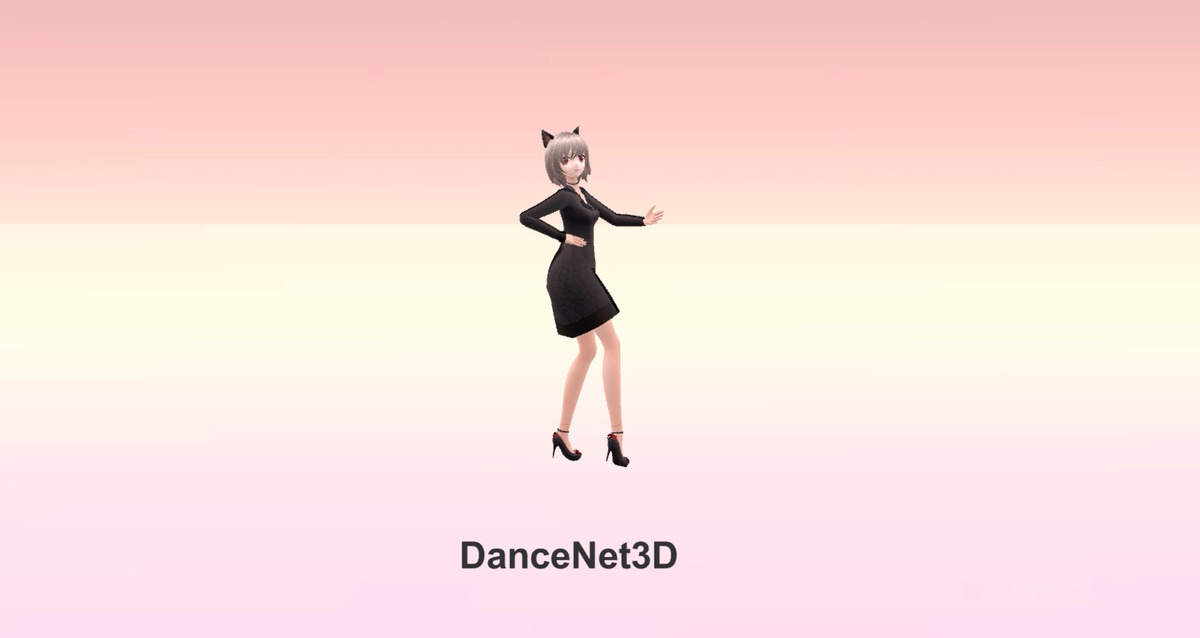
Overview
In contrast to previous works that define the problem as generation of frames of motion state parameters,
we formulate the task as a prediction of motion curves between key poses, which is inspired by the animation industry practice.
The proposed framework, named DanceNet3D, first generates key poses on beats of the given music and then predicts the in-between motion curves.
DanceNet3D adopts the encoder-decoder architecture and the adversarial schemes for training.
The decoders in DanceNet3D are constructed on MoTrans, a transformer tailored for motion generation.
In MoTrans we introduce the kinematic correlation by the Kinematic Chain Networks, and we also propose the Learned Local Attention module to take the temporal local correlation of human motion into consideration.
Furthermore, we propose PhantomDance, the first large-scale dance dataset produced by professional animatiors, with accurate synchronization with music.
Extensive experiments demonstrate that the proposed approach can generate fluent, elegant, performative and beat-synchronized 3D dances,
which significantly surpasses previous works quantitatively and qualitatively.
Paper Link
Project Link
Results
Demo Videos
Comparison
Dataset
The PhantomDance Dataset is realeased now! Please visit [https://github.com/libuyu/PhantomDanceDataset] for the details of the dataset and toolkit.